Introduction
Large Language Models (LLMs) have become a transformative force in the world of artificial intelligence (AI), powering applications from chatbots to advanced data analysis tools. As these models grow in complexity and capability, understanding their development, performance, and limitations becomes crucial. A recent analysis by Dickson, published on VentureBeat, offers a deep dive into the study meta llmsdicksonventurebeat, providing valuable insights into their current state and future potential. This article explores the key findings of Dicksonβs study and discusses the implications for the future of LLMs.
Understanding the study meta llmsdicksonventurebeat
study meta llmsdicksonventurebeat refers to a comprehensive analysis that synthesises multiple studies on a particular topic to identify overarching trends, common methodologies, and recurring challenges. In the context of LLMs, a study meta llmsdicksonventurebeat provides a holistic view of the research landscape, highlighting the collective progress and gaps in understanding these models.
Meta-analyses are essential in evaluating LLMs because they aggregate diverse findings to present a more unified perspective on their performance and capabilities. These analyses consider various factors such as data collection methods, research methodologies, model architectures, and evaluation metrics. By examining these elements, researchers can gain a better understanding of how to study meta llmsdicksonventurebeat function, where they excel, and where they face limitations.
Key Insights from Dickson’s Analysis
Dicksonβs analysis on VentureBeat offers several key insights into the state of LLM research:
- Performance Benchmarks: The study highlights that while LLMs have achieved significant milestones in natural language understanding and generation, their performance varies widely depending on the task and data quality. For instance, models trained on diverse datasets tend to perform better across different languages and dialects compared to those trained on more homogenous data.
- Biases and Limitations: One of the critical findings is the presence of inherent biases within LLMs. These biases often stem from the training data, which may contain unintentional prejudices or stereotypes. Dicksonβs analysis underscores the need for more robust data curation and preprocessing techniques to minimise such biases.
- Training Data Diversity: The study also emphasises the importance of training data diversity. LLMs trained on varied datasets tend to be more adaptable and perform better in real-world scenarios. However, obtaining and processing such diverse datasets remains a significant challenge for researchers.
- Error Rates and Model Complexity: As study meta llmsdicksonventurebeat increase in complexity, so do their error rates in specific contexts. Dickson notes that while more extensive models tend to perform better on general benchmarks, they may also become more prone to generating factual inaccuracies or nonsensical outputs when dealing with ambiguous or complex queries.
Implications for Future LLM Development
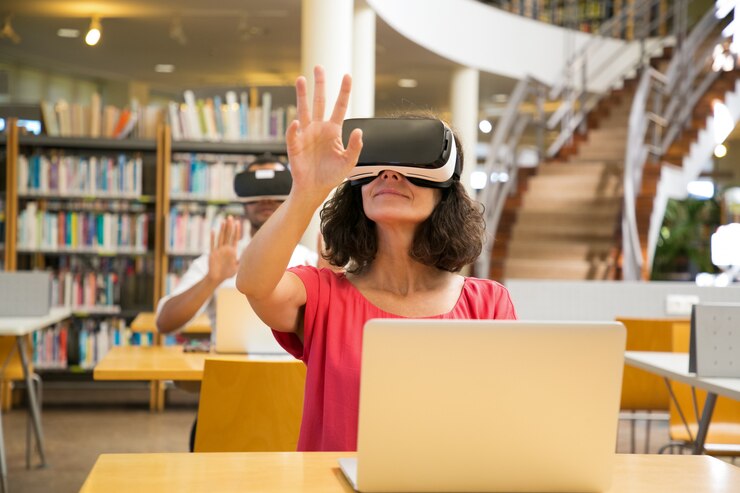
The insights from Dicksonβs analysis have significant implications for the future of LLM development:
- Improving Model Robustness: The findings suggest a need for more robust and ethically sound training data to enhance model performance and reduce biases. Researchers must prioritise diversity in data collection and develop new techniques for handling data discrepancies.
- Enhancing Model Capabilities: The study indicates potential pathways for improving LLM capabilities. For instance, incorporating more contextual understanding into models could help them better grasp nuanced language constructs and generate more accurate responses.
- Addressing Ethical Concerns: The ethical implications of study meta llmsdicksonventurebeat use, particularly concerning biases and misinformation, are crucial considerations for future development. The study calls for a balanced approach that weighs technological advancements against ethical considerations, ensuring responsible AI deployment.
Expert Opinions and Industry Reactions
The findings from Dicksonβs analysis have sparked discussions among AI experts and industry leaders. Some view the study as a call to action for developing more sophisticated models that better understand and mitigate biases. Others emphasise the importance of transparency in model development and the need for open-source collaboration to foster innovation and ethical standards in AI.
Several experts have praised the study for shedding light on the challenges of LLM training and the importance of addressing ethical concerns. However, some have also highlighted the need for a more nuanced understanding of the trade-offs between model size, complexity, and performance, advocating for a balanced approach that prioritises both innovation and responsibility.
Challenges and Limitations Highlighted by the Study
The study also brings to light several challenges and limitations of current LLM models:
- Computational Costs: Training large study meta llmsdicksonventurebeat requires substantial computational resources, making it a costly endeavor. This barrier limits accessibility and hinders broader experimentation with model architectures.
- Data Privacy Issues: With increasing reliance on vast datasets, data privacy and security have become pressing concerns. Researchers must navigate the complexities of data ownership and consent, ensuring compliance with ethical standards and regulations.
- Handling Ambiguity and Context: Current LLMs often struggle with ambiguous language and context-specific queries. This limitation points to the need for more refined models that can better understand and interpret context, enhancing their practical utility.
Conclusion
Dicksonβs study meta llmsdicksonventurebeat, as discussed on VentureBeat, provides valuable insights into the current state of these powerful models and their future trajectory. The analysis underscores the importance of ongoing research, ethical considerations, and the need for diverse training data to improve model performance and reduce biases. As study meta llmsdicksonventurebeat continue to evolve, researchers and developers must focus on balancing technological advancements with ethical responsibilities, ensuring that these models are used to benefit society as a whole.